Summary
A Fortune 500 government contractor partnered with Striveworks to predict lightning-induced wildfires from a range of multisource data. The team developed a data fusion model to blend the National Oceanic and Atmospheric Administration (NOAA) Geostationary Lightning Mapper (GLM) data with raster imagery and weather data. When changes in real-world weather data led to model failure, Striveworks’ machine learning operations (MLOps) helped helped quickly remediate the model, successfully delivering a workflow to consistently process 96 hours of multisource data in 25 minutes to optimize firefighters’ mission planning.
Accuracy
Minutes end to end, from data processing to automated report delivery
Predictions in six weeks
Lightning Destroys Millions of Acres of Forest Every Year
Lightning is the leading cause of wildfires in non-tropical forests,1 responsible for destroying 4,206,960 acres in 2022 alone.2 Current best practices for wildfire identification are ineffective for large-scale prevention. The United States has over 751 million acres of forest—much too large an area for firefighters to inspect each lightning strike location.3 Strikes also routinely spark small ignitions that smolder for several days before smoke becomes visible.
Data Fusion Supports Firefighting by Predicting Lightning-Induced Wildfires
To save critical time in wildfire identification, a Fortune 500 government contractor partnered with Striveworks to use data from the NOAA GLM to predict lightning-induced wildfires.
Depending on environmental and atmospheric conditions, each lightning strike has a range of potential to ignite a wildfire. The project team combined GLM data with raster imagery and tabular weather data to track over 150 features in an AI model for predicting the risk of wildfires. Because these datasets varied by data type and resolution, data fusion was necessary. Models for this project were built and deployed using the Striveworks MLOps platform.
The project proceeded according to plan, and the model performed well—until the winter and spring of 2022/2023, when the area under observation experienced unusually wet conditions. These conditions resulted in a phenomenon known as model drift: when a model that once performed well begins to respond poorly in real-world conditions.
Remediation Restores a Failing Model to High Performance
The team commenced remediation, starting with identifying potential failure modes. They soon identified the cause of model failure: Due to the change in weather, incoming data was now out of distribution with the model’s training data. To resolve the problem, the team sourced a new dataset within the range of the incoming data and then trained a new model on it using the Striveworks MLOps platform. Once returned to production, this updated model exceeded all customer goals, including F1 score (a measure of predictive performance), accuracy, and recall (also known as the true positive rate).
Goal |
Actual |
|
F1 score (peak 14-day moving average performance) |
0.65 |
0.80+ |
Accuracy |
0.85 |
0.87+ |
Recall |
0.65 |
0.90+ |
96 Hours of Data Aggregated in 25 Minutes Supports Firefighting Missions
The effects were immediate. The team used this model consistently to aggregate, process, exploit, and deliver a report on 96 hours of multisource data in just 25 minutes—a capability that is functionally impossible otherwise. The entire process was automated to deliver this analysis to firefighters just in time for daily mission planning meetings so that they can focus their limited resources on scouting the areas that are most likely to develop into a blaze.
Ultimately, this project reaffirmed the critical importance of remediation for sustaining the performance of machine learning (ML) models as they face the fluctuations in real-world data. It also underscores the Striveworks approach to ML post-production, including the continuous monitoring of production models that makes rapid remediation possible.
1 “Lightning Is the Leading Cause of Wildfires in Boreal Forests, Threatening Carbon Storage,” PreventionWeb, November 9, 2023, https://www.preventionweb.net/news/lightning-leading-cause-wildfires-boreal-forests-threatening-carbon-storage.
2 “Lightning-Caused Wildfires,” National Interagency Fire Center, accessed June 20, 2024,
https://www.nifc.gov/fire-information/statistics/lightning-caused.
3 “Forester’s Blog: So, How Much Forest Is There in the U.S. and Canada?”, Sustainable Forestry Initiative Blog, August 9, 2022,
https://forests.org/so-how-much-forest-is-there-in-the-us-and-canada/.
Data fusion is a methodology that combines data from multiple disparate sources to create a more nuanced, accurate insight. Fusion is challenging, as each data source may have a distinct format, structure, quality, or other characteristic that impedes standardization. It requires large amounts of configuration and data handling as well as heavy computational resources.
The wildfire detection project fused the following data types to predict the likelihood of lightning-induced fires:
- Tabular data from the NOAA GLM
- Three-dimensional and time-series data from the NOAA
- Raster imagery of forest cover
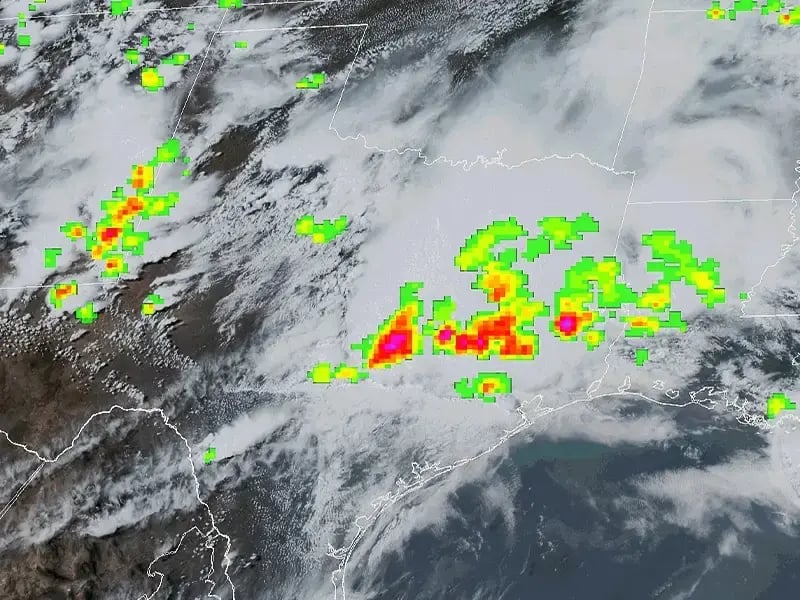
Related Resources

How Geospatial Machine Learning Transforms Decision-Making (With 7 Use Cases)
Get an overview of seven use cases of how events happening on the Earth’s surface open a world of opportunities for organizations to get ahead of problems and improve their processes.