Geospatial Machine Learning Transforms Decision-Making (7 Use Cases)
This blog post is part of a series on geospatial analysis. View the other posts here:
Geospatial analysis is a suite of methodologies that leverages geolocation to inform and drive decision-making. By understanding events happening on the Earth’s surface, organizations open a world of opportunities to get ahead of problems and improve their processes.
Machine learning takes geospatial analysis even further. Using computer vision models, organizations can scale up their data processing and make it more granular—automating most workflows and unleashing capabilities that are impossible without artificial intelligence.
From the military to firefighters, the Department of Transportation to financial markets, more and more organizations are pairing geospatial data with machine learning to get incredible results. Here, we look at some of the most exciting use cases for geospatial machine learning happening today.
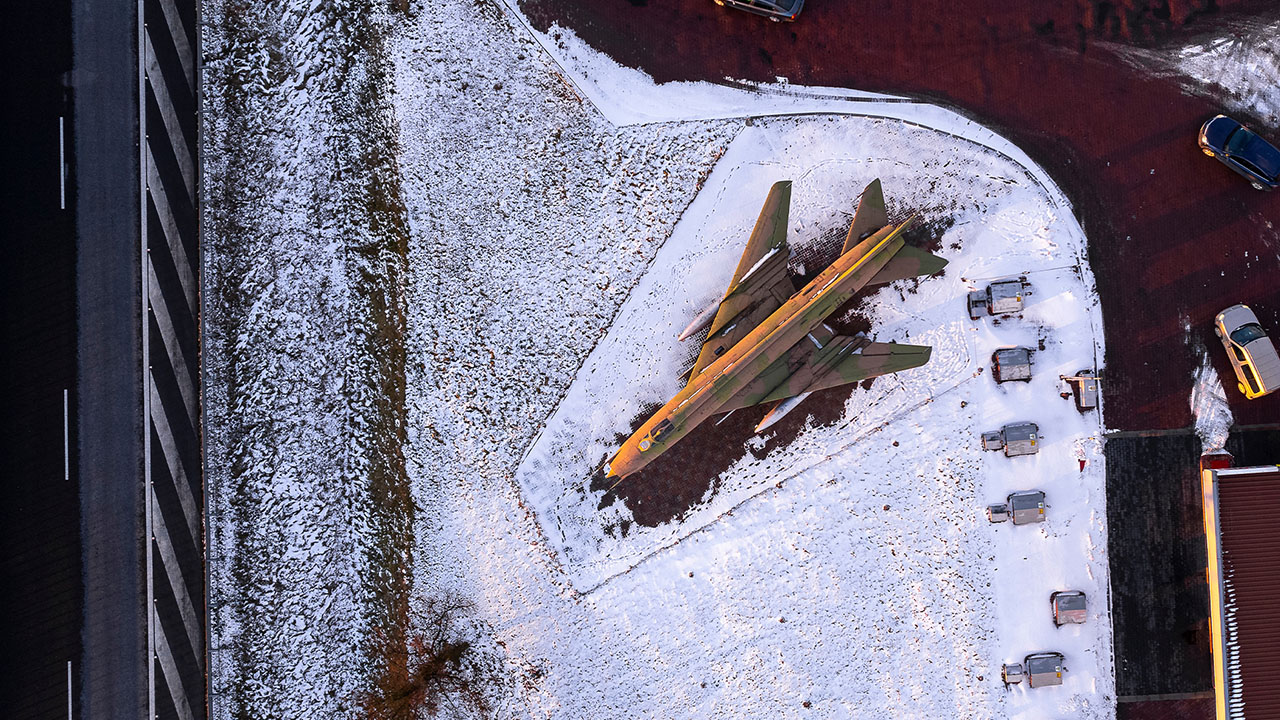
Use Case 1: Identifying Military Aircraft Anywhere on Earth
The military are among the savviest consumers of geospatial data.
“Defense and intelligence is the most mature market for geospatial data,” says Brett Foreman, senior product manager at Capella Space, a Striveworks partner and leading provider of synthetic aperture radar (SAR) data, which uses a moving radar antenna to produce high-resolution images regardless of weather or nighttime conditions.
Because the stakes are so high for every military decision, armed forces have invested heavily in technologies that give them rich information about the world. That investment resulted in the creation of the National Geospatial-Intelligence Agency (NGA), formed in 1996 to collect and analyze geospatial data for national security purposes.
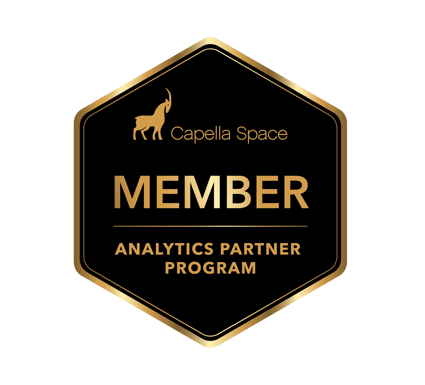
NGA analysts regularly use geospatial data to explore active war zones, potential areas of vulnerability, and ways soldiers could avoid detection from the land, sea, and air. This data is critical for operations and defense planning, particularly when it comes to understanding infrastructure and resources held by rivals.
“A classic geospatial use case is understanding the type and number of aircraft at different airfields,” says Foreman.
“Aircraft have a certain amount of range to them, and they also have certain things that they carry. Knowing the quantity and type of aircraft at different places around the world is really important for intelligence officials who are planning how to respond to their competitors and adversaries.”
Machine learning scales up and accelerates this work. The NGA has enormous volumes of geospatial data available for military operations—too much for analysts to review manually. Object detection models that run on these datasets can identify aircraft by type in seconds, speeding up intelligence gathering and empowering the military with a full picture of their targets. Indeed, the advantage that machine learning gives the NGA has led the agency to call out computer vision and artificial intelligence as key parts of its 2023 strategy.
No-code technologies make it even easier to get results. One Striveworks defense customer used the Striveworks machine learning operations platform to train an object detection model without any code and then deployed the model to find objects on its first day running.
The customer saw the immediate possibilities for geospatial machine learning, saying, “I’m excited about what we can do.”
Use Case 2: Predicting the Next Big Wildfire
Wildfires are a threat to people and the environment that seem to spring up without warning. Each year, thousands of wildfires burn millions of acres across the United States, causing death, injury, and displacement of people fleeing the blazes. In particular, wildfires sparked by lightning strikes cause an overwhelming amount of destruction: 4,206,960 acres in 2022 alone. Fortunately, geospatial data can help.
One Fortune 500 company is using Striveworks’ strength with geospatial data to predict the locations of lightning-caused wildfires before they turn serious. A machine learning model in the Striveworks platform fuses geospatial data with rasterized data and tabular data to predict lightning-caused fires just after the lightning strikes—a feat that analysts struggle to perform on their own.
The volume of data involved in these predictions overwhelms analysts’ abilities to review it manually. By using machine learning, the project team can handle the huge amount of data processing, including daily fluctuations in storms that spark wide variability in lightning.
With Striveworks, the company has been able to collect and process 96 hours of data into a report in less than 25 minutes, giving users the most up-to-date information to help extinguish these fires before they spread.
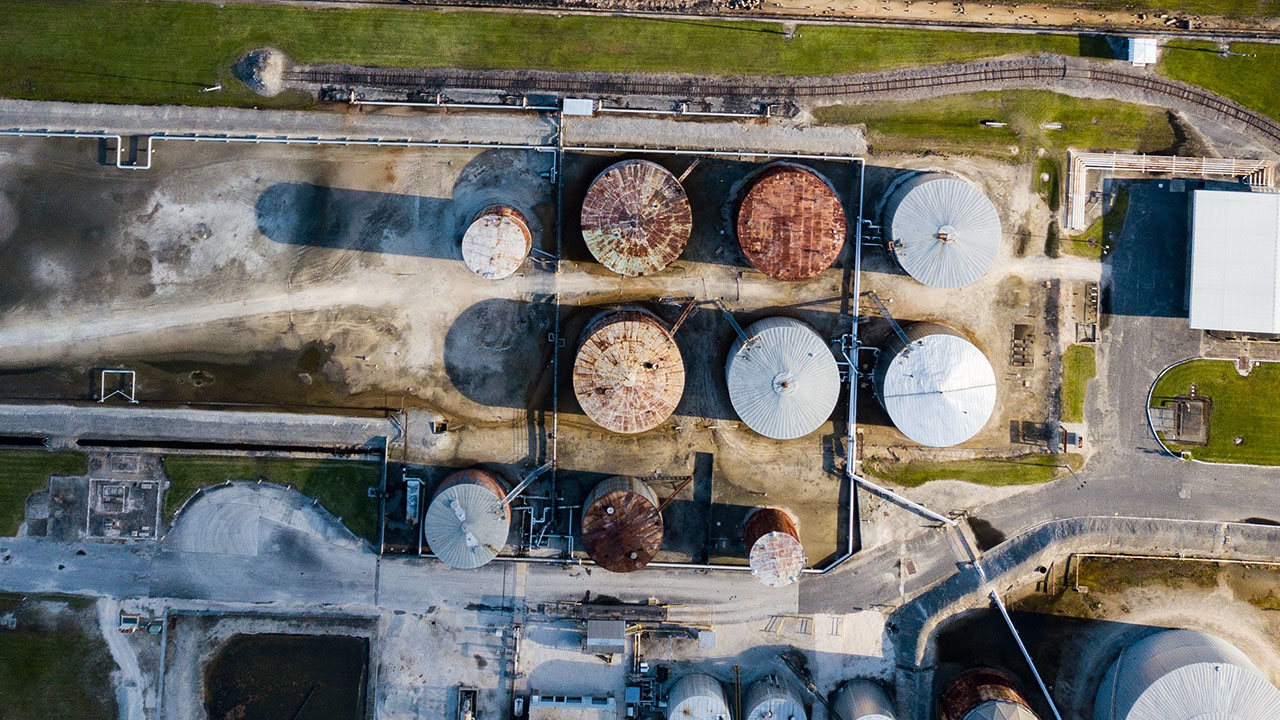
Use Case 3: Trading More Profitably, Thanks to Better Data
Modern satellites can take extremely high-resolution images of the Earth on a regular basis, which adds wholly new capabilities for geospatial analytics.
One way people are using this geospatial data is for insights that affect commodities trading.
“If you take pictures of every country’s major terminals on some reasonable cadence, then you can develop a pretty good estimate of above-ground oil storage.”
— Brett Foreman, Capella Space
“A classic [use case] that’s been quite successful is measuring the quantity of oil in the world’s above-ground oil storage tanks,” says Foreman.
“Basically, these tanks have a floating lid, so you can tell by the length of the shadow how close the lid is to the top or the bottom. If you know the size of the gigantic barrel, then you can figure out how much oil is in there.
“If you take pictures of every country’s major terminals on some reasonable cadence, then you can develop a pretty good estimate of above-ground oil storage.”
Commodities traders can use this information to forecast the price of oil in advance of regulators publishing official storage volumes. They can then trade futures and options with this extra information.
Similarly, people have used geospatial imagery to track supply chain activity that could affect stock pricing. “There’s a whole blog that looks at Tesla’s parking lots, where they store completed vehicles, to see how many vehicles were completed, so they can measure if they’re behind their forecast or in front of their forecast,” says Foreman. Investors have used similar tactics with McDonald’s, Lowe’s, Wal-Mart, and other retailers.
Of course, observing slight changes across image after image is difficult and time-consuming. A machine-learning-based change detection algorithm can handle the hard work of tracking the shifts in shadows at key terminals, giving traders the time-sensitive edge they need to trade profitably.
Use Case 4: Assessing Risk and Damage for Insurance Providers
Insurance companies are also strong consumers of geospatial data.
“Insurers use overhead imagery to detect the features of a property, the important characteristics for underwriting insurance,” says Foreman. “That would be your roof type, pitch, whether there’s standing water or staining—generally, indicators of what’s at the property and, to a certain extent, the condition of those key elements.”
This information is critical for insurance companies, who face big losses if policies are priced incorrectly or if risk factors, such as a property being located in a floodplain, are not captured ahead of time.
“If you can combine your detection of the extent of the standing water with a good knowledge of the terrain from another data source, then you can kind of do the volumetric to say, How high is the water?”
— Brett Foreman, Capella Space
Insurers also use geospatial imagery to assess the extent of damage after a catastrophe.
“Capella Space and other Earth observation imagery providers do quite a bit of work in the insurance claims line of business,” says Foreman. “For us, we focus on flooding: Where was the flooding? Did it impact certain structures? If you can do that on a broad scale quickly, then you can speed up the claims process and help people get checks faster.”
Foreman notes that Capella’s SAR imagery shows the presence of water especially well, but to get a full understanding of flood damage, additional data sources are needed. Machine learning is able to fuse data from weather stations and other sources with SAR imagery to produce a more granular, accurate understanding of flood damage.
“If you can combine your detection of the extent of the standing water with a good knowledge of the terrain from another data source, then you can kind of do the volumetric to say, ‘How high is the water?’”
Use Case 5: Predicting and Tracing Disease Outbreaks
Roughly 15 million people die from infectious diseases like tuberculosis and malaria every year.
Public health authorities have a long history of using geospatial data in their work to halt the spread of these diseases. (In fact, the first application of geospatial analysis mapped London during the cholera epidemic of 1854.) By drawing connections between known outbreak areas and common mobility patterns, public health authorities can project which other areas are at risk.
Geospatial data has seen heavy use by epidemiologists in tracking the spread of COVID-19. The WHO uses this technology to identify concentrations of people over 65 years of age, as well as common outbreak locations for contact tracing. With this information, doctors and nurses can target their interventions at areas and people that need them most.
Striveworks’ partner Esri has devoted an entire section of its website to coronavirus response, including resources on mapping confirmed cases, infection tracing over time, vulnerable populations, and facilities, staff, or supplies needed to mitigate the effects of the disease.
Machine learning takes disease tracing to another level. Models can discover patterns among large sets of multisource data that humans struggle to understand on their own, such as cell phone tower signals, rideshare routes, and traffic at pharmacies.
Infectious diseases are especially dynamic, so even the best modeling risks becoming stale. Machine learning tools that scan model outputs for accuracy are invaluable for ensuring that straying models get remediated and that insights still help health authorities respond to outbreaks promptly and effectively.
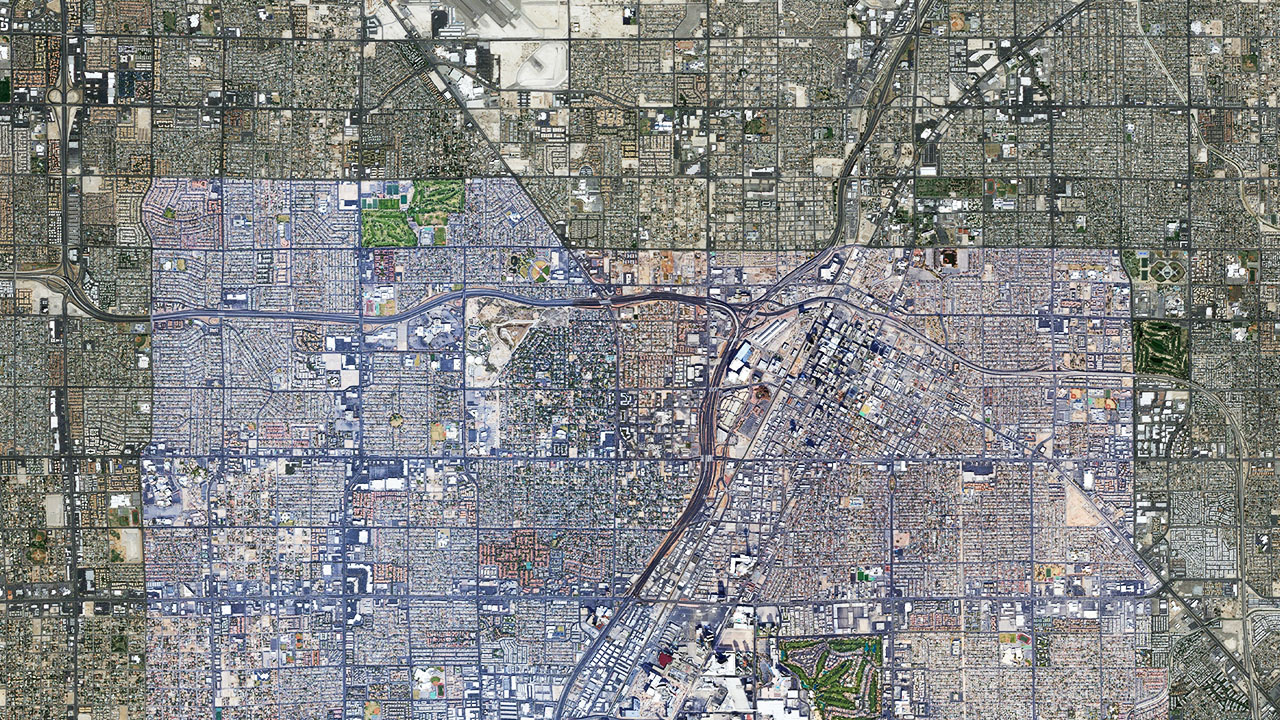
Use Case 6: Finding Dangerous Intersections to Prevent Car Crashes
The Nevada Department of Transportation (DOT) is using geospatial data to save lives in a different way: plotting high-risk intersections that are responsible for an outsize number of traffic collisions.
All DOTs maintain standard maps of their roadways. By overlaying geospatial data about car crashes on these maps, the Nevada DOT has figured out which stretches of road in the state suffer the most collisions. With this information, the DOT can tell which areas pose the greatest risk to motorists and then explore ways to address the problem. They can also use this data to predict areas that may need interventions in the future.
Machine learning can make this process even more informative and efficient. It enables analysts to process and analyze much larger volumes of data than they can manually. By bringing together geospatial data from multiple state and local sources into a comprehensive data warehouse, transportation authorities can use machine learning to discover especially deadly intersections across entire states or regions. They can also learn more about factors that contribute to traffic collisions and their level of importance, such as weather or road conditions.
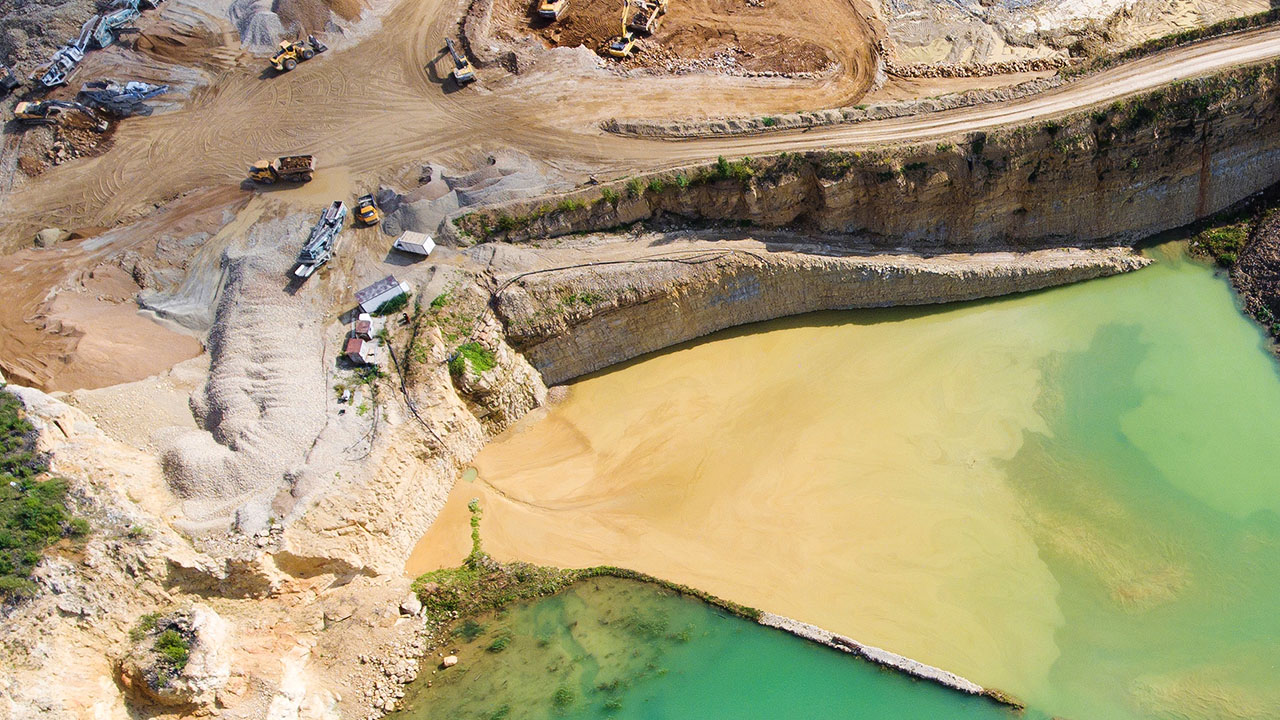
Use Case 7: Keeping Mining Operations Safe and Productive
Mining companies use geospatial data for many parts of their operation—from prospecting to mine planning to risk management during active excavation.
Because the business of mining is so closely tied to the Earth, geospatial imagery weighs heavily in mining companies’ decisions. It contains critical information for miners, such as the location of mineral deposits, characteristics of ore bodies, and relevant features of the terrain that may affect operations.
BHP is a major user of geospatial data, laying the groundwork for its current program with Esri in the early 2000s. It assesses imagery to understand geological structures of mineable areas and to scout potential mineral deposits in remote locations where other methods of prospecting may struggle.
Mines use aerial imagery to track the environmental impact of their activities, as well as land use and water resource management. They can also use it to identify safety risks, as geospatial analysis can spot issues with pit wall stability or the integrity of tailings dams that are less visible at ground level.
“Many operators are starting to use satellite imagery alongside traditional aerial imagery to more efficiently monitor activity and potential risks across mining sites that are commonly less accessible and cover broad areas,” says Foreman. “SAR satellite imagery specifically provides an added advantage of accurately monitoring land displacement and ground deformation—conditions that can increase safety risks and costs to the mining operators.”
Image segmentation is an obvious use for geospatial machine learning in the mining industry. A computer vision model can easily identify interesting features of the Earth’s surface, such as signs of ore bodies or deposits of hard rock that may require a different approach to excavation. A model can rapidly scan imagery and tip analysts for important objects to review.
What’s the Next Geospatial Machine Learning Use Case That Will Change the World?
The combination of geospatial data and machine learning is transforming the world around us. While some applications of geospatial machine learning are obvious, others are completely novel and redefine the boundaries of what’s possible. These use cases show how innovative leaders are using the power of these technologies to solve problems and extend their capabilities—from shifting advantages in financial markets to saving lives from disease, war, and traffic collisions.
More than anything, these geospatial machine learning use cases show how much potential there is when you pair an innovative technology, like computer vision, with a rich source of data. As geospatial data increases in resolution in the coming years, and as more and more organizations get familiar with machine learning, even more novel use cases will emerge.
It really raises the question: What incredible ways are you going to use geospatial machine learning to change the world?
Do you have a new use case for geospatial machine learning? Reach out to discuss how Striveworks can help.